One more run! The algorithms driving the gig economy
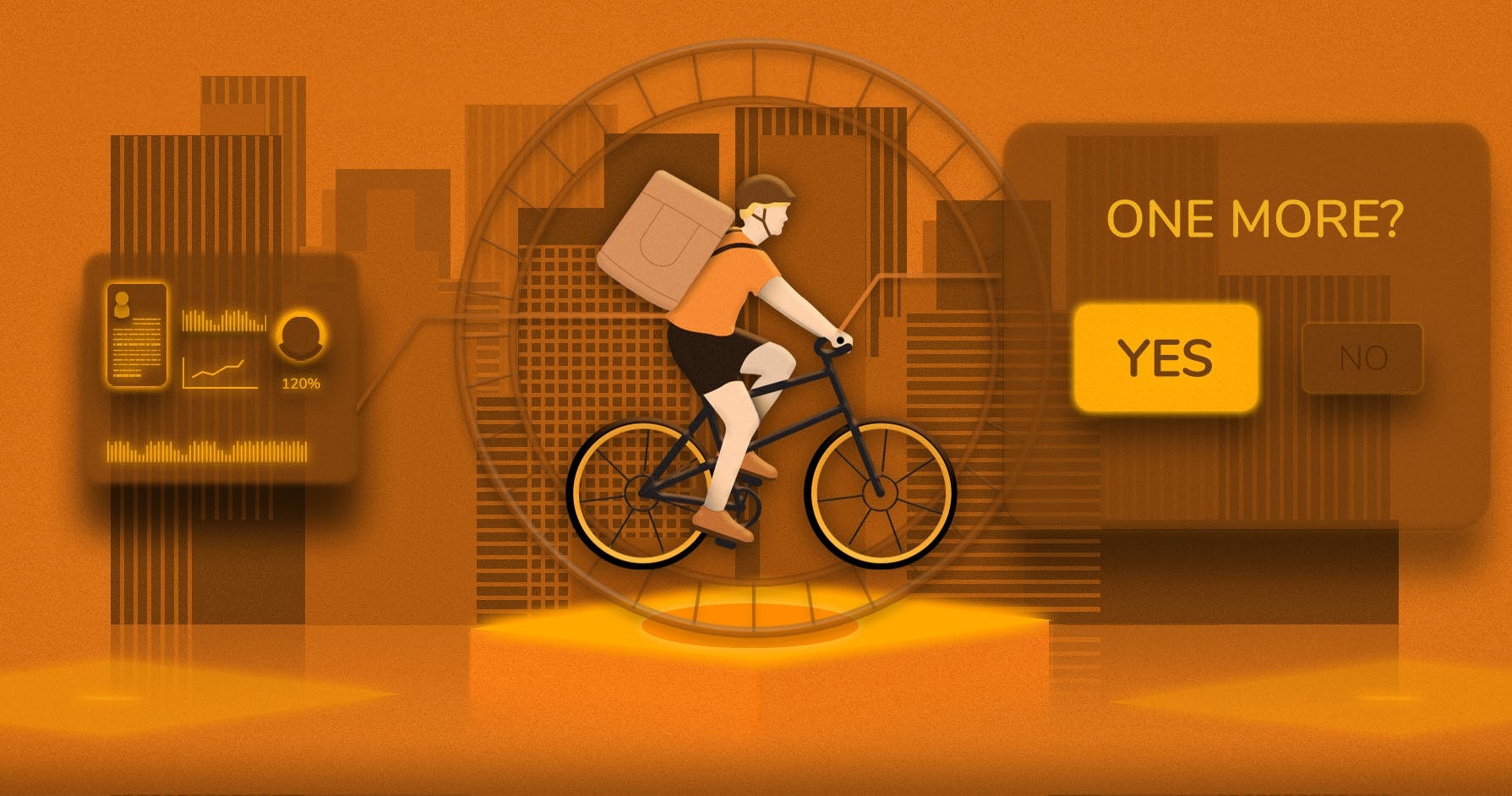
TOPICS
Virtual EconomyIn university, I made deliveries for Uber Eats as a side gig. After several hours, I was tired, but the app constantly pushed me to do “one more run,” which often turned into an extra hour.
This got me thinking about what so-called “gig economy” apps are doing when they nudge workers to work just a little bit more.
L’Atelier defines the gig economy as “a marketplace for excess supply of time. It facilitates the generation of supplemental income through tasks and opportunistic informal labour such as transport, cleaning, running errands, or clerical work through digital platforms.” In this piece, I'll examine how the gig economy is structured (using Uber as a primary example), how its strategic use of technology contributes to its success, and what those things convey about the realities of gig work.
Gig economy work is autonomous and relatively well-paid, making it a convenient option for people from all kinds of backgrounds: All you need is a valid ID and a smartphone to start working. For people in non-standard situations, like immigrants, part-time workers or students, it provides a foothold in the job market and the ability to gain valuable skills along the way.
People entering the gig economy are attracted to the idea of freelancing or entrepreneurship. They are often motivated by the desire to define their own revenue goals, controlling the time and effort they invest in their work, and not being accountable to an employer. Thus platforms like Uber promote independence as the cornerstone of workers’ careers, which is key to the model’s success.
Once inside the model, users typically manage their workload through a gamified app, which employs psychological levers to trigger mental reward systems—like that “one more run” alert I mentioned earlier.
By default, the driver-side version of Uber’s mobile application approves the next run before drivers even finish the current one. Interrupting the flow requires extra effort (and potentially distracts from driving), leveraging our natural bias towards inertia, largely in favour of the platform. Researchers liken this system to Netflix’s auto-play button, which is useful ... but can also contribute to unintended binge-watching.
This gets problematic when gig workers grow addicted to the rush and become disinclined to stop, even for things as minor as bathroom breaks, as Uber representatives have stated. When gig work becomes an addiction, non-working hours get coloured by impatience and withdrawal, yielding negative consequences on their psyches and personal lives. No matter the nature of gig work, workers may feel like they can’t turn down a job that the algorithm offers to them, which conflicts with marketing narratives of freedom and independence.
From a platform perspective, the gig economy’s model of non-standard labour conditions is beneficial. Not having to provide a shared workplace or equipment allows them to save up to 30 percent in expenses, compared to traditional employment. Remote workers are also more productive than on-site colleagues: They tend to work harder and for longer hours, disproving the received belief that they are less productive.
These corporate benefits are compounded by the algorithms these companies so often employ. Algorithms dispatch gigs between workers according to complex (and opaque) calculations. The surrounding narrative portrays this as creating productivity gains by perfectly matching supply to demand, a theoretical win-win situation that favours both the employer and workers.
Uber has an ambivalent strategy for reassuring stakeholders and, in the long run, maximising profit. With attractive incentives—like initial high hourly compensations, the rhetoric of independence, or giveaways like its “$500 sign-up bonus”—it seeks to constantly grow its workforce. Therefore it can trigger competition between drivers to dramatically increase platform responsiveness and client satisfaction.
But over time, drivers encounter progressively more frustrating working conditions. Platforms grant bonuses for higher pay, but these ultimately depend on the number of runs the algorithm assigns to them. This in turn relies on opaque methods for dispatching runs to those algorithmically considered most relevant to completing a commission, based on location and customer reviews.
The precariousness of gig workers, and the lack of alternatives (top gig apps work similarly to each other), gives platforms the freedom to set compensations to their advantage. When the app does not automatically assign a new job even before you've finished, waiting for a new commission can take an average of 20 minutes per hour—one-third of workdays, amounting to days that can be up to 16 hours long to barely reach minimum wage. Per research from the OECD, 19 percent of full-time gig workers are below the poverty line, versus only three percent for standard workers.
To make this look sustainable, a lot happens behind the scenes of the gig economy. Uber and its competitors have invested significantly in PR campaigns to convince the general public and investors that their growth is synonymous to tech innovation and economic liberation.
By “investing in growth,” each company wants to create a network effect. In this theory, a company creates a system where it’s more convenient or worthwhile for users—customers and restaurants in this case—to choose it, in terms of diversity of choice and low prices, thus cultivating a monopoly and enabling them to raise customer-facing costs.
However, economic theory tells us such models mostly work to repay fixed costs, which are in fact very low in the Uber model: Only the costs of developing phone apps can be considered as such, since gig platforms don’t have to provide equipment or physical workspaces to workers.
Marketing costs, like the incentives Uber grants couriers (in the form of bonuses and subsidies), are actually what costs the company the most, and this investment rises as the fleet grows. This disproportionate investment in new gig workers impacts compensation for existing ones. The American Affairs Journal observes that “almost all of Uber’s margin improvement since 2015 is explained by this reduction of driver compensation down to minimum wage levels, not by improved efficiency.” Uber also engaged in various accounting schemes to present yearly losses as growth investments.
While Uber is a useful example of current gig economy models, it is not the only company that engages in these tactics. Its competitors do the same: “Comparable cuts at Lyft resulted in a labor-capital wealth transfer of $1 billion,” which inevitably impacted workers' earnings.
The gig economy model, paired with innovations like these gamified algorithms, is seducing more and more sectors of the economy. Relying on people in precarious situations to engage in service work has been around a long time; the only novelty is that now technology can optimise this by leveraging cognitive biases.
At scale, and considering the long-term effects on both individuals and the economy at large, are we willing to perpetuate that just to have sushi delivered to our doorsteps?
13 Jan 2022
-
Olivier Lhuissier
Illustrations by Dominika Haas.
DATA-DRIVEN TECH & SOCIAL TRENDS. DISCOVERED WEEKLY. DELIVERED TO YOUR INBOX.
02/03
Related Insights
03/03
L’Atelier is a data intelligence company based in Paris.
We use advanced machine learning and generative AI to identify emerging technologies and analyse their impact on countries, companies, and capital.